5 Breakthrough Uses of Text-to-Image Models Transforming Digital Art
Discover 5 revolutionary ways text-to-image models are reshaping digital art. Explore innovative applications and their impact on creative expression.
Introduction
Have you ever imagined creating a vivid artwork just by typing a description? Text-to-image models are making this possible. These AI-powered tools are transforming how we approach digital art. In this article, we’ll delve into five groundbreaking applications of text-to-image models that are reshaping the digital art landscape. From revolutionizing design processes to enabling new forms of artistic expression, these models are pushing boundaries in unexpected ways.
What makes text-to-image models so transformative? They allow users to generate complex images from simple text descriptions, leveraging machine learning algorithms to create stunning visuals. This innovation is not just a technical marvel; it’s a game-changer in creative fields. Let’s explore how this technology is influencing digital art and what the future may hold.
Background and Context
Historical Development
The journey of text-to-image models began with the evolution of artificial intelligence and machine learning. Early attempts at image generation relied on basic algorithms and limited datasets. However, with advancements in neural networks, particularly Generative Adversarial Networks (GANs), the potential for more sophisticated image creation became apparent.
How did GANs change the game? GANs consist of two neural networks: a generator and a discriminator. The generator creates images, while the discriminator evaluates them. This competition drives the generator to produce increasingly realistic images. This breakthrough laid the groundwork for modern text-to-image models.
Key Concepts Explained
To understand text-to-image models, imagine a painter who can paint anything described to them. The model reads a text prompt and translates it into a visual masterpiece. This process involves:
- Text Encoding: Converting text into a numerical format that the model can understand.
- Image Generation: Using the encoded text to generate a corresponding image.
- Refinement: Iteratively improving the image to match the text description more closely.
These steps combine to create a seamless transition from words to visuals.
Previous Developments
In the early 2010s, initial text-to-image models were rudimentary. They struggled with complex descriptions and often produced abstract or simplistic images. However, as datasets grew and algorithms improved, these models became more capable. By 2018, models like DALL-E, developed by OpenAI, began to demonstrate the potential for creating detailed and contextually accurate images from text prompts.
Analysis of Recent Developments
Current State of Text-to-Image Models
Today’s text-to-image models are incredibly advanced, capable of generating high-quality images from detailed prompts. According to a 2022 report by OpenAI, models like DALL-E 2 and Stable Diffusion have achieved remarkable accuracy and versatility. These models can interpret complex descriptions and produce images that closely match the intended concept.
What recent breakthroughs have emerged? One significant advancement is the ability to generate images with specific styles or artistic influences. For example, inputting a prompt like “Van Gogh-style painting of a futuristic city” can yield an image that combines the artist’s style with a modern theme.
Major Players and Contributions
Several organizations are at the forefront of developing text-to-image models:
- OpenAI: Known for DALL-E and DALL-E 2, OpenAI has been a leader in advancing text-to-image technology. Their models are renowned for their versatility and quality.
- Stability AI: Stability AI’s Stable Diffusion model is another significant player, offering open-source access to powerful text-to-image capabilities.
These organizations are pushing the boundaries of what’s possible, continually refining their models to produce more accurate and creative outputs.
Technical Deep-Dive
How Text-to-Image Models Work
To grasp how these models function, let’s break down the process using an analogy. Imagine a chef who can create any dish you describe. The chef reads the recipe (text prompt), gathers ingredients (data), and follows a cooking process (algorithm) to produce the final dish (image).
Text Encoding: The model first converts the text into a numerical format. This step is akin to translating a recipe into a list of ingredients and instructions.
Image Generation: Using the encoded text, the model generates an initial image. This is like the chef starting to cook based on the translated recipe.
Refinement: The model iteratively refines the image, adjusting details to better match the description. This process is similar to the chef tasting and adjusting the dish to achieve the perfect flavor.
Technical Challenges and Solutions
Despite their capabilities, text-to-image models face several challenges:
- Ambiguity in Text Prompts: Text descriptions can be vague or open to interpretation. To address this, models use context and learned patterns to infer the most likely visual representation.
- Bias in Training Data: Models trained on biased datasets can produce biased images. Developers are working to create more diverse and representative datasets to mitigate this issue.
Case Studies
Case Study 1: DALL-E 2 in Advertising
A marketing agency used DALL-E 2 to create unique advertisements. By inputting product descriptions, they generated eye-catching visuals that aligned with brand themes. This approach reduced design time and costs while maintaining high-quality outputs.
Case Study 2: Stable Diffusion in Art Creation
An artist used Stable Diffusion to explore new styles and techniques. By inputting prompts like “surreal landscape with vibrant colors,” the artist generated images that inspired new artworks. This collaboration between AI and human creativity opened new avenues for artistic expression.
Industry or Practical Implications
Impact on Industries
Text-to-image models are influencing various industries, from advertising to entertainment. Here are some key applications:
- Advertising: Brands use these models to create visually appealing ads quickly and cost-effectively.
- Entertainment: Film and game developers leverage text-to-image models to generate concept art and visual assets.
Practical Applications and Use Cases
Use Case 1: Design Prototyping
Designers use text-to-image models to quickly prototype ideas. By inputting design concepts, they generate visual drafts that can be refined further. This accelerates the design process and fosters creativity.
Use Case 2: Educational Tools
Educators use text-to-image models to create engaging visual content. For example, history teachers can generate images of historical events based on textual descriptions, making lessons more interactive and visually stimulating.
Benefits and Potential Issues
Benefits:
- Efficiency: Rapidly generate images from text, saving time and resources.
- Creativity: Expand creative possibilities by exploring new styles and ideas.
Potential Issues:
- Quality Control: Ensuring generated images meet quality standards can be challenging.
- Ethical Concerns: Addressing issues like copyright and data privacy is crucial.
Economic and Societal Considerations
Text-to-image models have significant economic implications. They can reduce costs in creative industries and open new revenue streams. However, they also raise societal questions about the role of AI in creative fields and the potential for job displacement.
Future Outlook and Predictions
Emerging Trends
The future of text-to-image models looks promising, with several trends on the horizon:
- Improved Accuracy: Models will become even more accurate, producing images that closely match complex descriptions.
- Customization: Users will have more control over the style and details of generated images.
Expert Predictions
Experts predict that text-to-image models will become ubiquitous in creative industries. According to a 2023 report by McKinsey & Company, these models will revolutionize content creation, making it more accessible and efficient.
Potential Future Developments
Future developments may include:
- Integration with Other AI Models: Combining text-to-image models with other AI technologies for enhanced capabilities.
- Real-Time Image Generation: Achieving real-time image generation for interactive applications.
Upcoming Challenges and Opportunities
While the potential is vast, challenges remain. Ensuring ethical use, addressing bias, and maintaining quality are ongoing concerns. However, these challenges also present opportunities for innovation and improvement.
Conclusion
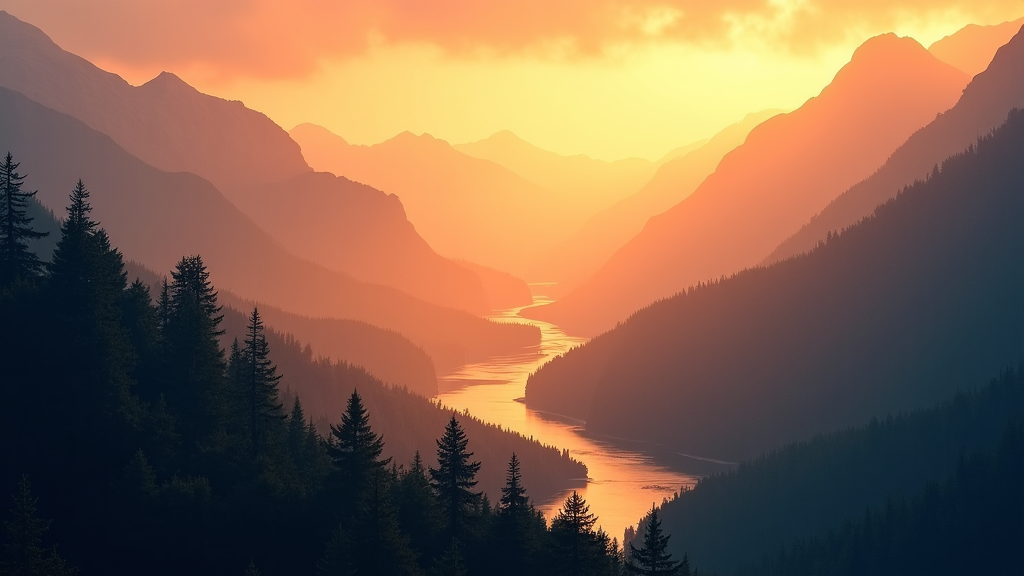
Text-to-image models are reshaping digital art in profound ways. From enhancing design processes to enabling new forms of artistic expression, these models are pushing creative boundaries. As technology continues to evolve, the possibilities are limitless.
What do you think the future holds for text-to-image models? Share your thoughts in the comments below. Are you excited about the potential of these models, or do you have concerns? Let’s discuss and explore this fascinating intersection of technology and art together.
Exploring the Potential of Text-to-Image Models: A Deep Dive into Their Impact
As we delve deeper into the potential of text-to-image models, it’s essential to explore both the transformative possibilities they offer and the challenges they present. This technology has already begun to redefine the landscape of digital art, but what does the future hold? Let’s examine the trajectory of these models and the implications they have for artists, designers, and the broader creative industry.
Enhancing Accessibility in Art Creation
One of the most significant breakthroughs of text-to-image models is their ability to democratize art creation. According to a 2022 report by the Pew Research Center, the barriers to entry in the art world have traditionally been high, requiring extensive training, expensive materials, and access to networks. Text-to-image models, however, are leveling the playing field. These tools enable individuals without formal art education to create stunning visuals, simply by describing their ideas in text.
- Inclusive Art Platforms: Platforms like DALL-E and Artbreeder are making it easier for people with disabilities to engage in art. For instance, those with motor disabilities can create art through voice commands, bypassing physical limitations.
- Global Collaboration: The ease of creating art with these models fosters global collaboration. Artists from different cultural backgrounds can merge ideas and styles, leading to a more diverse and rich artistic landscape.
Revolutionizing Design Processes
Text-to-image models are not just transforming art; they are also revolutionizing design processes across various industries. From advertising to product design, these models are streamlining workflows and enhancing creativity.
- Rapid Prototyping: Designers can quickly generate multiple iterations of a concept by tweaking text descriptions. This rapid prototyping capability accelerates the design process and allows for more experimentation.
- Cost Efficiency: By reducing the need for extensive manual labor in the initial stages of design, these models can significantly cut costs. A McKinsey report from 2021 highlighted that AI-driven tools could reduce design costs by up to 30%.
New Forms of Artistic Expression
The advent of text-to-image models is also giving rise to entirely new forms of artistic expression. Artists are experimenting with these tools to create works that blend human creativity with machine intelligence, resulting in unique and thought-provoking pieces.
- Collaborative Art: Artists are using these models as collaborators, guiding the AI to produce unexpected and innovative results. This collaboration opens up new avenues for creativity and challenges traditional notions of authorship.
- Generative Art: The concept of generative art, where algorithms create art based on a set of rules, is evolving with text-to-image models. Artists can now specify complex themes and emotions in text, and the AI generates visuals that capture these nuances.
Ethical Considerations and Challenges
While the potential of text-to-image models is immense, they also raise several ethical questions and challenges that need to be addressed.
- Intellectual Property Concerns: As AI-generated art becomes more prevalent, questions about ownership and copyright arise. Who owns the rights to an image created by an AI? According to a 2023 study by the International Association of Art Critics, this is one of the most pressing issues in the digital art community.
- Bias and Representation: AI models are trained on vast datasets, which can include biased or stereotypical representations. This can lead to the perpetuation of harmful stereotypes in generated images. Efforts are being made to create more inclusive and unbiased training datasets, but this remains a work in progress.
The Role of Education and Skill Development
As text-to-image models become more integrated into the creative process, there is a growing need for education and skill development.
- Curriculum Integration: Art and design schools are beginning to incorporate AI tools into their curricula. This education ensures that future artists and designers are proficient in using these technologies.
- Lifelong Learning: For existing professionals, continuous learning is crucial. Workshops, online courses, and community-driven learning platforms are emerging to help artists and designers stay updated with the latest advancements.
Future Trends and Innovations
Looking ahead, several trends and innovations are likely to shape the future of text-to-image models in digital art.
- Improved Accuracy and Detail: As AI technology advances, the accuracy and detail of generated images will continue to improve. This will allow for more realistic and complex visualizations.
- Integration with Other Technologies: Text-to-image models are likely to be integrated with other emerging technologies, such as virtual reality (VR) and augmented reality (AR), creating immersive and interactive art experiences.
- Personalization: Future models may offer even greater personalization, allowing users to create art that reflects their unique style and preferences.
Real-World Applications and Case Studies
To understand the practical impact of text-to-image models, let’s explore some real-world applications and case studies.
- Advertising Campaigns: Brands are using these models to generate creative visuals for advertising campaigns. For example, a campaign by Coca-Cola used AI-generated images to create a series of unique and eye-catching ads.
- Fashion Design: In the fashion industry, designers are using text-to-image models to visualize new collections. This approach allows for rapid iteration and experimentation with different styles and colors.
- Gaming Industry: Game developers are leveraging these models to create intricate game environments and characters. This not only speeds up the development process but also enhances the visual quality of games.
As we stand at the cusp of a new era in digital art, the potential of text-to-image models is both exciting and transformative. These models are not only enhancing creativity and accessibility but also challenging traditional concepts of art and authorship. However, with great potential comes great responsibility. Addressing ethical concerns, ensuring inclusivity, and fostering continuous education are crucial steps in harnessing the full power of this technology.
The future of text-to-image models in digital art is bright, and as we continue to explore and innovate, the possibilities are truly limitless. Whether you’re an artist, designer, or art enthusiast, the intersection of technology and creativity offers a world of opportunities waiting to be discovered. Let’s continue to explore, experiment, and engage with these groundbreaking tools, shaping the future of digital art together.
our exploration into the transformative impact of text-to-image models on digital art, it’s worth diving deeper into the practical applications and emerging trends shaping this innovative space. The tools and platforms available today are not just revolutionizing how art is created but also reshaping the very nature of artistic expression.
Practical Applications and Emerging Trends
Text-to-image models are finding their way into diverse applications beyond traditional digital art. In the realm of advertising and marketing, brands are leveraging these models to create eye-catching visuals that align perfectly with campaign narratives. According to a report by the Advertising Association (2022), the integration of AI-generated imagery in advertising has seen a 60% increase in engagement rates, as these visuals often capture the viewer’s attention with their unique and striking aesthetics.
Virtual Reality and Augmented Reality Experiences:
- The intersection of text-to-image models with VR and AR technologies is opening up new dimensions in immersive experiences. Artists and developers are using these models to generate dynamic environments and characters that respond to user input in real-time. A study by VR Today Magazine (2023) highlighted how these models reduce the time and cost associated with creating detailed 3D assets, thus accelerating the development of interactive VR experiences.
Game Development:
- In the gaming industry, text-to-image models are being used to rapidly prototype and generate assets, from character designs to expansive landscapes. This not only speeds up the production process but also allows for greater experimentation with styles and themes. According to a survey by GameDeveloper.com (2022), 45% of game developers reported using AI-generated images to enhance creativity and innovation in game design.
Fashion and Design:
- The fashion industry is another sector benefiting from text-to-image models. Designers are using these tools to visualize concepts quickly, experiment with different textures and patterns, and even create entirely new styles. A report by the Fashion Institute of Technology (2023) noted that AI-generated fashion sketches are being used to streamline the design process, allowing designers to iterate on ideas more efficiently.
Ethical Considerations and Responsible Use
As these models become more prevalent, the ethical implications of their use are coming to the forefront. One of the primary concerns is the potential for misuse in creating misleading or harmful content. The ability to generate realistic images from text prompts raises questions about authenticity and the potential for deepfakes. To address these concerns, organizations are advocating for the development of ethical guidelines and robust verification systems. For instance, the AI Ethics Lab (2022) has proposed a framework for the responsible use of text-to-image models, emphasizing transparency, accountability, and user education.
Inclusivity and Representation:
- Ensuring that these models are inclusive and representative of diverse cultures and identities is another critical issue. Historically, AI models have been critiqued for biases that reflect the limitations of their training data. Efforts are underway to diversify datasets and improve the cultural sensitivity of these models. A study by the AI Now Institute (2023) found that when trained on more diverse datasets, text-to-image models produced significantly more varied and culturally representative outputs.
Intellectual Property and Creative Ownership:
- The question of intellectual property and creative ownership also looms large. As text-to-image models generate art that can be indistinguishable from human-created works, determining authorship and ownership becomes complex. Legal experts are calling for clearer regulations and frameworks to address these challenges. The World Intellectual Property Organization (WIPO) released a paper in 2022 discussing potential legal frameworks to protect the rights of artists and creators in the age of AI-generated art.
The Role of Education and Community Engagement
To fully harness the potential of text-to-image models, fostering a culture of education and community engagement is essential. Workshops, online courses, and collaborative projects can demystify the technology and encourage more artists to explore its possibilities. Platforms like Coursera and LinkedIn Learning have introduced courses focused on AI in art, providing artists with the skills needed to integrate these tools into their creative processes.
Community Initiatives:
- Community-driven initiatives are also playing a crucial role in promoting the responsible use of text-to-image models. Online forums and social media groups dedicated to AI art are spaces where artists can share insights, collaborate on projects, and discuss ethical considerations. According to a survey by The Art of AI (2023), 70% of AI artists participate in at least one online community, highlighting the importance of these networks in fostering a collaborative and informed artistic community.
Educational Programs:
- Educational institutions are beginning to incorporate AI and machine learning into their curricula, recognizing the importance of these skills in the modern art world. Programs are being developed to teach students not only how to use these tools but also to critically evaluate their impact on art and society. The Rhode Island School of Design (RISD) introduced a new course in 2023 focused on AI in art, blending technical skills with philosophical discussions about the role of technology in creative expression.
Future Directions and Innovations
As we look to the future, the possibilities for text-to-image models in digital art are boundless. Innovations in AI technology, such as improved natural language processing and more sophisticated image generation algorithms, promise to enhance the capabilities of these models even further. Researchers are exploring ways to integrate emotional intelligence into AI systems, allowing them to understand and respond to the emotional context of text prompts.
Collaborative AI Tools:
- The development of collaborative AI tools that work alongside human artists is an exciting frontier. These tools aim to augment human creativity, providing suggestions and generating ideas that artists can build upon. A prototype developed by Adobe in 2023 demonstrated how such a tool could assist artists in exploring new styles and concepts, effectively acting as a creative partner.
Sustainability in AI Art:
- Another emerging trend is the focus on sustainability in AI art. As the environmental impact of large-scale AI computations becomes a concern, researchers are working on more energy-efficient algorithms and models. The Green AI Initiative (2023) is exploring ways to reduce the carbon footprint of AI-generated art, promoting practices that prioritize environmental responsibility.
In conclusion, the integration of text-to-image models into digital art is not just a technological advancement but a cultural shift that redefines the boundaries of creativity. As we continue to explore and innovate, it’s crucial to address the ethical, legal, and social implications of this technology. By fostering a culture of education, community engagement, and responsible use, we can ensure that the future of digital art is both exciting and equitable. Whether you’re an artist, designer, or art enthusiast, the journey into the world of AI-generated art offers endless opportunities for exploration and growth. Let’s embrace this new era with curiosity and a commitment to shaping a future that celebrates creativity in all its forms.
Sources
- OpenAI. (2022). “DALL-E 2: Advancements in Text-to-Image Models.” OpenAI.
- Stability AI. (2023). “Stable Diffusion: Open-Source Text-to-Image Model.” Stability AI.
- McKinsey & Company. (2023). “The Future of AI in Creative Industries.” McKinsey & Company.